Mastering Machine Learning Data Labeling: Enhance Your AI Models
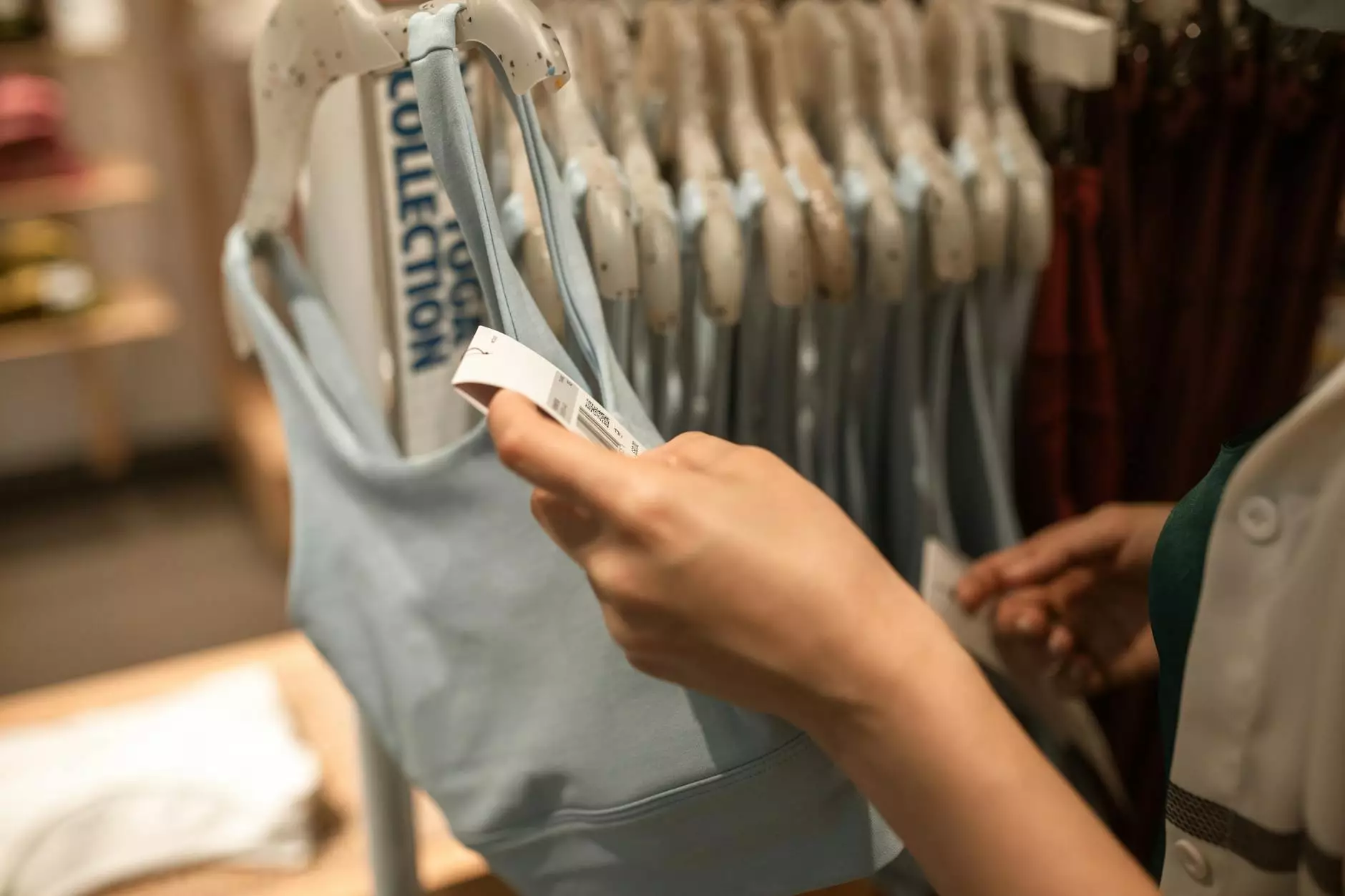
In the evolving landscape of technology, machine learning has emerged as a pivotal element in driving advancement across various industries. At the heart of this technology lies the concept of data labeling — a crucial process that underlies the ability of machine learning algorithms to learn and make predictions. This article dives deep into the realm of machine learning data labeling, highlighting its significance, methodologies, tools, and best practices to ensure your AI systems achieve remarkable accuracy and efficiency.
The Significance of Machine Learning Data Labeling
Data is the lifeblood of machine learning. However, not just any data will do; it must be accurately labeled to train effective models. Here are some key reasons why machine learning data labeling is vital:
- Quality of Predictions: Labeled data allows models to distinguish between different classes and features, enhancing their predictive capabilities.
- Bias Reduction: Proper labeling can reduce the risk of biases in machine learning models, leading to fairer outcomes.
- Applicability Across Industries: From healthcare to finance, accurate labeling is indispensable for training models that cater to specific industry needs.
Understanding the Data Labeling Process
The machine learning data labeling process involves several interrelated steps that ensure your data is prepared for model training. Below is a breakdown of this essential process:
1. Data Collection
The first step involves gathering a diverse dataset that reflects the real-world scenarios your model is intended to operate in. The quality and volume of the collected data are critical for effective training.
2. Data Annotation
Data annotation, often referred to as data labeling, is where the magic happens. This is where raw data is tagged or labeled with descriptive information. For example:
- Image Data: Bounding boxes, segmentation masks, and classifications.
- Text Data: Sentiment labels, entity recognition tags, and topic classifications.
- Audio Data: Transcriptions, emotion tags, and speaker labels.
3. Model Training
With your labeled data in hand, it’s time to train your machine learning model. The model learns to make predictions based on the labeled examples provided during this phase.
4. Evaluation and Iteration
Post-training, the model needs to be evaluated to assess its accuracy and performance using a separate validation dataset. Depending on the outcomes, you may need to iterate on your labeling and training processes.
Popular Methods for Data Annotation
When it comes to machine learning data labeling, several methodologies exist, each with its advantages and applications. Here are a few popular methods:
- Manual Annotation: Human annotators label the data, ensuring accuracy and context understanding. Although it's time-consuming, it's often necessary for complex datasets.
- Automated Annotation: Utilizing algorithms and pre-trained models to label data can speed up the process, but it may lack the precision that human annotators provide.
- Crowdsourcing: This method leverages a wide pool of individuals to complete labeling tasks. Platforms like Amazon Mechanical Turk allow businesses to label large datasets efficiently.
Challenges in Data Labeling
While machine learning data labeling is essential, it comes with its own set of challenges:
- Quality Control: Ensuring high-quality labels is crucial. Inconsistent or inaccurate labeling can severely hinder model performance.
- Scalability: As datasets grow, scaling labeling efforts can be overwhelming without the right tools and resources.
- Time Consumption: Labeling large datasets is often a time-intensive process, particularly if done manually.
The Role of Data Annotation Tools
To overcome the challenges in machine learning data labeling, various tools have been developed to streamline the annotation process. KeyLabs offers robust solutions in this arena, featured as:
KeyLabs Data Annotation Tool
The KeyLabs Data Annotation Tool is designed to simplify the labeling process, enhancing efficiency and accuracy. Here are some features that set it apart:
- Intuitive User Interface: The platform is user-friendly, allowing annotators to work efficiently without steep learning curves.
- Collaboration Features: Team members can collaborate in real-time, ensuring a consistent labeling standard across projects.
- Support for Multiple Data Types: Whether it’s images, text, or audio, KeyLabs caters to diverse data formats.
KeyLabs Data Annotation Platform
The KeyLabs Data Annotation Platform takes label management a step further by integrating robust tools for large-scale labeling needs:
- Artificial Intelligence Integration: Leverage AI-assisted labeling to enhance speed while maintaining accuracy.
- Workflow Customization: Tailor workflows to meet unique project demands, facilitating smoother operations.
- Analytics Dashboard: Monitor labeling progress and quality metrics to make informed decisions quickly.
Best Practices for Effective Data Labeling
To maximize the potential of your machine learning models, consider implementing the following best practices in your data labeling efforts:
- Define Clear Guidelines: Provide detailed labeling instructions to annotators to maintain consistency across the dataset.
- Regular Training for Annotators: Ongoing training for annotators ensures that they are up-to-date with the latest practices and maintain high standards of quality.
- Feedback Loop: Establish a feedback mechanism where annotators can learn from mistakes, improve, and discuss ambiguities in labeling.
- Use High-Quality Sample Datasets for Testing: Prior to full-scale labeling, test the guidelines on a smaller dataset to refine the process.
- Incorporate Quality Control Measures: Regularly conduct spot-checks of labeled data to identify inconsistencies and improve processes.
The Future of Machine Learning Data Labeling
The evolution of machine learning data labeling is exponential, with innovations constantly reshaping the space. Moreover, the following trends are expected to dominate the future:
- Increased Automation: The rise of AI technologies will continue to automate repetitive tasks, enhancing efficiency while preserving quality.
- Enhanced Collaboration: With more teams embracing remote work, collaborative data labeling platforms will become essential for effective project management.
- Focus on Ethical AI: There will be a greater emphasis on addressing biases in datasets, requiring precise labeling practices to promote fair and responsible AI.
Conclusion
In the age of artificial intelligence, the importance of machine learning data labeling cannot be overstated. As businesses strive to leverage big data for predictive analytics and optimization, investing in effective data labeling processes becomes critical. With the right tools, methodologies, and best practices, organizations can significantly enhance the performance of their machine learning models, leading to innovative solutions that drive growth and efficiency. Explore how KeyLabs can empower your data annotation efforts and ensure your AI solutions reach their full potential.
Ultimately, embracing the complexities and challenges of data labeling will pave the way for groundbreaking advancements across industries. Prepare your business for the future of AI with the best practices in machine learning data labeling, and watch as your models transform raw data into actionable insights.