Unlocking the Power of Open Source Image Annotation Tools
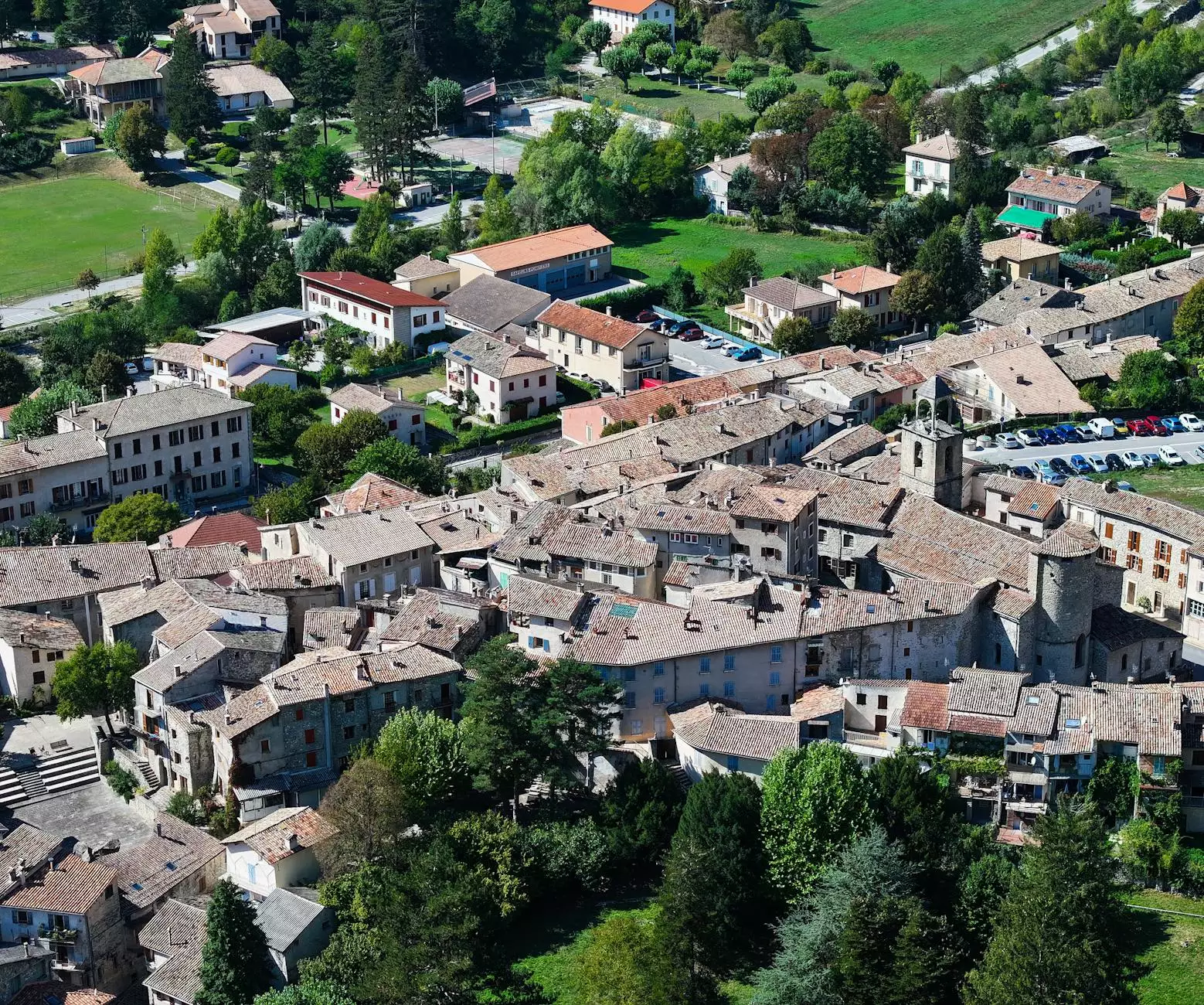
The digital age has ushered in an explosion of data, and annotations play a pivotal role in harnessing this information effectively. Within this vast realm lies the niche of image annotation—a vital process for labeling images, necessary for training machine learning models in various domains such as computer vision and artificial intelligence. Among the various solutions available, open source image annotation tools have gained significant attention for their flexibility, adaptability, and community-driven innovation. This article delves deeply into the world of open source image annotation tools, showcasing how they empower businesses and enhancing productivity in data annotation processes.
What is Image Annotation?
Image annotation refers to the technique of labeling or tagging images with relevant metadata so that machines can understand and process visual data. The technique combines human intelligence and machine learning, laying the foundation for applications ranging from self-driving cars to facial recognition software. By marking specific components within images—such as objects, landmarks, and elements—users train AI models to recognize these patterns autonomously.
The Importance of Image Annotation in Business
Businesses across various industries rely heavily on accurate image annotations to enhance their products and services. Here are some of the key benefits:
- Enhancing Computer Vision Models: High-quality labeled datasets allow for the development of robust computer vision applications.
- Improving User Experience: Annotation aids in improving user interfaces and enhancing user interaction through features like image categorization.
- Streamlining Workflows: Automated processes powered by machine learning can save significant time and resources.
- Supporting Marketing Efforts: Annotated images can aid in targeted advertising and customer segmentation strategies.
Why Choose Open Source Image Annotation Tools?
Open source tools present a compelling alternative to commercial software. They offer numerous benefits that can greatly impact your organization's efficiency and cost-effectiveness:
1. Cost-Effectiveness
One of the most striking advantages of open source image annotation tools is their cost-effectiveness. Unlike their proprietary counterparts, they are often available at no charge, significantly reducing overhead costs associated with data annotation projects.
2. Customizability
Open source tools can be tailored to meet specific business needs. Developers can modify the source code, integrate new features, or customize existing functionalities to create a tool that fits their workflows perfectly. This flexibility is particularly advantageous for companies with unique operational requirements.
3. Community Support and Collaboration
Using open source software allows businesses to tap into a vibrant community of developers and users. This collaborative environment fosters innovation, as users contribute improvements, report bugs, and share best practices, leading to continual development and refinement of the tools.
4. Transparency and Security
Open source tools provide enhanced transparency. Organizations can review the code to ensure there are no backdoors or vulnerabilities, fostering trust in the system's integrity. Additionally, they can contribute to the code's security, benefiting from collective oversight.
Features of a High-Quality Open Source Image Annotation Tool
When selecting an open source image annotation tool, you will want to consider the following crucial features:
- User-Friendly Interface: A simple and intuitive interface reduces the learning curve for team members.
- Robust Annotation Capabilities: Look for tools that support various types of annotations, including bounding boxes, polygonal segmentation, and semantic segmentation, to meet diverse project needs.
- Collaboration Tools: Features that allow multiple users to annotate images concurrently can greatly enhance productivity and efficiency.
- Exporting Options: The ability to export annotations in various formats facilitates seamless integration with machine learning workflows.
- Compatibility: Ensure that the tool integrates smoothly with your existing software and systems for streamlined processes.
Top Open Source Image Annotation Tools
The following are some of the leading open source image annotation tools that can transform your data annotation projects:
1. LabelImg
LabelImg is a popular image annotation tool that allows users to annotate images with bounding boxes. It supports both PASCAL VOC and YOLO formats, making it easy to use with machine learning frameworks. The tool's straightforward interface allows for rapid labeling, which is essential for projects with large datasets.
2. VGG Image Annotator (VIA)
The VGG Image Annotator (VIA) is another robust tool catered toward annotating images with an easy-to-navigate interface. It's entirely browser-based, allowing users to upload images and annotate them without complex installations. VIA supports multiple annotation types, including keypoints, polygons, and bounding boxes, making it versatile for various use cases.
3. RectLabel
RectLabel is specifically designed for Mac users, providing functionalities such as bounding box annotation, pixel-wise segmentation, and even video labeling support. It stands out for its excellent usability and integration with TensorFlow and Keras, making it a go-to choice for developers in those ecosystems.
4. Supervisely
Supervisely offers a comprehensive platform that not only provides image annotation tools but also supports data management and model training. It features an extensive library of pre-built models and integrations with popular deep learning frameworks, making it an excellent choice for career data scientists looking to streamline their workflows.
How to Implement an Open Source Image Annotation Tool
Implementing an open source image annotation tool involves several key steps:
1. Define Your Requirements
Before selecting a tool, define the specific needs of your project. Consider the types of annotations required, the scale of your data, collaboration needs, and the technical expertise of your team.
2. Choose the Right Tool
Based on your requirements, evaluate the available open source options. Look for tools that align with your team's skill levels and the complexity of your projects.
3. Train Your Team
Once you’ve selected a tool, train your team members on how to use it effectively. Many open source tools come with extensive documentation and user guides that can greatly assist in the learning process.
4. Start Annotating
Begin the annotation process. Set clear guidelines for consistency and quality assurance to ensure the labeled data is reliable.
5. Evaluate and Iterate
Regularly evaluate the annotation process and make adjustments as needed. Gather feedback from your team to improve workflows continually.
Conclusion
In conclusion, open source image annotation tools offer businesses an essential resource for tackling the challenges posed by data annotation. They provide cost-effective, customizable, and transparent solutions that can enhance efficiency and output quality in machine learning projects. As the demand for labeled data continues to grow across the business landscape, leveraging the power of these tools can be a game-changer for organizations aiming to stay ahead in the competitive market. By adopting open source image annotation tools, businesses can not only streamline their processes but also inspire innovation and collaboration within their teams, ensuring they remain at the forefront of technological advancement.
Explore the vibrant world of open source image annotation tools today and unlock your business's potential with Keylabs.ai!
image annotation tool open source